ICCDE 2026 Keynote Speakers
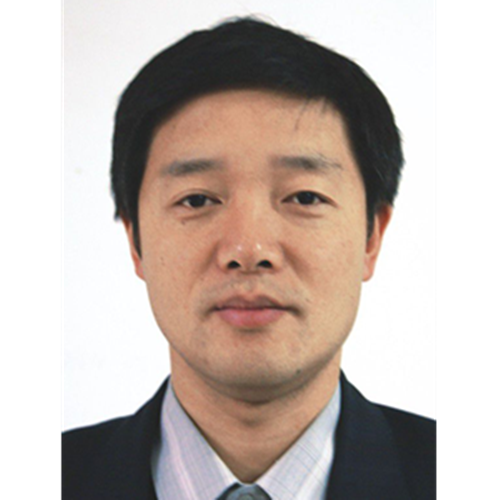
Prof. Hai Jin (IEEE Fellow)
Huazhong University of Science and Technology, China
Hai Jin received the PhD degree in computer engineering from Huazhong University of Science and Technology, China in 1994. He is a Cheung Kung scholars chair professor of computer science and engineering with Huazhong University of Science and Technology. In 1996, he was awarded a German Academic Exchange Service fellowship to visit the Technical University of Chemnitz in Germany. He worked with The University of Hong Kong between 1998 and 2000, and as a visiting scholar with the University of Southern California between 1999 and 2000. His research interests include computer architecture, virtualization technology, cluster computing and cloud computing, peer-to-peer computing, network storage, and network security. He was awarded Excellent Youth Award from the National Science Foundation of China in 2001. He is the chief scientist of ChinaGrid, the largest grid computing project in China, and the chief scientists of National 973 Basic Research Program Project of Virtualization Technology of Computing System, and Cloud Security. He has co-authored 22 books and published more than 700 research papers. He is a fellow IEEE, CCF, and a life member of the ACM.
ICCDE Previous Keynote Speakers
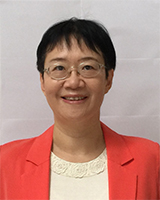
Prof. Ling Liu (IEEE Fellow)
Georgia Institute of Technology, USA
Ling Liu is a full professor
in the School of Computer Science at Georgia
Institute of Technology. She directs the
research programs in the Distributed Data
Intensive Systems Lab (DiSL), examining various
aspects of big data systems and analytics. Prof.
Liu is an elected IEEE Fellow, a recipient of
IEEE Computer Society Technical Achievement
Award (2012), and a recipient of the best paper
award from numerous top venues, including IEEE
ICDCS, WWW, ACM/IEEE CCGrid, IEEE Cloud, IEEE
ICWS. Prof. Liu served on editorial board of
over a dozen international journals, including
the editor in chief of IEEE Transactions on
Service Computing (2013-2016). Currently, Prof.
Liu is the editor in chief of ACM Transactions
on Internet Computing (since 2019). She is the
chair of IEEE CS Fellow Evaluation Committee
(FY2024). Her current research is primarily
supported by National Science Foundation, CISCO
and IBM.
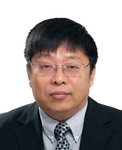
Prof. Derong Liu (IEEE Fellow)
University of Illinois at Chicago, USA
Derong Liu received the Ph.D. degree in electrical engineering from the University of Notre Dame in 1994. He was a Staff Fellow with General Motors Research and Development Center, from 1993 to 1995. He was an Assistant Professor with the Department of Electrical and Computer Engineering, Stevens Institute of Technology, from 1995 to 1999. He joined the University of Illinois at Chicago in 1999, and became a Full Professor of Electrical and Computer Engineering and of Computer Science in 2006. He was selected for the “100 Talents Program” by the Chinese Academy of Sciences in 2008, and he served as the Associate Director of The State Key Laboratory of Management and Control for Complex Systems at the Institute of Automation, from 2010 to 2015. He is now a Full Professor with the School of Automation, Guangdong University of Technology. He has published 19 books. He is the Editor-in-Chief of Artificial Intelligence Review (Springer). He was the Editor-in-Chief of the IEEE Transactions on Neural Networks and Learning Systems from 2010 to 2015. He received the Faculty Early Career Development Award from the National Science Foundation in 1999, the University Scholar Award from University of Illinois from 2006 to 2009, the Overseas Outstanding Young Scholar Award from the National Natural Science Foundation of China in 2008, and the Outstanding Achievement Award from Asia Pacific Neural Network Assembly in 2014. He is a Fellow of the IEEE, a Fellow of the International Neural Network Society, and a Fellow of the International Association of Pattern Recognition.
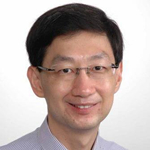
Prof. Kay Chen Tan (IEEE Fellow)
City University of Hong Kong, Hong Kong
Kay Chen Tan received the B.Eng. (First Class Hons.) degree in electronics and electrical engineering and the Ph.D. degree from the University of Glasgow, U.K., in 1994 and 1997, respectively. He is a Full Professor with the Department of Computer Science, City University of Hong Kong, Hong Kong SAR. He has published over 300 refereed articles and 10 books. Prof. Tan is the Editor-in-Chief of the IEEE Transactions on Evolutionary Computation (IF: 8.058), was the Editor-in-Chief of the IEEE Computational Intelligence Magazine from 2010 to 2013, and currently serves as the Editorial Board Member of over 10 journals. He is currently an elected member of IEEE CIS AdCom, an IEEE DLP Speaker, and a Changjiang Chair Professor in China.
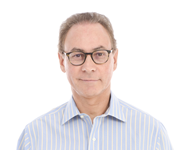
Prof. Peter Haddawy
Mahidol University, Thailand
Professor Haddawy received a BA in Mathematics from Pomona College in 1981 and MSc and PhD degrees in Computer Science from the University of Illinois-Urbana in 1986 and 1991, respectively. He was tenured Associate Professor in the Department of Electrical Engineering and Computer Science at the University of Wisconsin-Milwaukee, and Director of the Decision Systems and Artificial Intelligence Laboratory there through 2002. Subsequently, he served as Professor of Computer Science and Information Management at the Asian Institute of Technology (AIT) through 2010 and the Vice President for Academic Affairs from 2005 to 2010. He served in the United Nations as Director of UNU-IIST from 2010 through 2013. Professor Haddawy has been a Fulbright Fellow, Hanse-Wissenschaftskolleg Fellow, Avery Brundage Scholar, and Shell Oil Company Fellow. His research falls broadly in the areas of Artificial Intelligence, Medical Informatics, and Scientometrics and he has published over 130 refereed papers with his work widely cited. His research in Artificial Intelligence has concentrated on the use of decision-theoretic principles to build intelligent systems and he has conducted seminal work in the areas of decision-theoretic planning and probability logic. His current work focuses on intelligent medical training systems and application of AI techniques to surveillance and modeling of vector-borne disease. In the area of Scientometrics Prof. Haddawy has focused on development of novel analytical techniques motivated by and applied to practical policy issues. He currently holds a full professorship in the Faculty of ICT at Mahidol University in Thailand where he is Director of the Mahidol-Bremen Medical Informatics Research Unit and Deputy Dean for Research. He also holds an Honorary Professorship and the Chair for Medical Informatics at the University of Bremen in Germany.
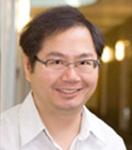
Prof. Chen-Huei Chou
College of Charleston, SC, USA
Chen-Huei Chou received the B.S. in Information and Computer Engineering from Chung Yuan Christian University, Taiwan, the M.S. in Computer Science and Information Engineering from National Cheng Kung University, Taiwan, the M.B.A. from the University of Illinois at Chicago, Chicago, Illinois, USA, and the Ph.D. in Management Information Systems from the University of Wisconsin-Milwaukee, Wisconsin, USA.
He is an Associate Professor of Information Management and Decision Sciences in the School of Business at the College of Charleston, SC, U.S.A. His research has been published in MIS journals and major conference proceedings, including MIS Quarterly, Journal of Association for Information Systems, Decision Support Systems, IEEE Transactions on Systems, Man, and Cybernetics, Computers in Human Behavior, Internet Research, and Journal of Information Systems and e-Business Management. His areas of interests include web design issues in disaster management, ontology development, Internet abuse in the workplace, text mining, data mining, knowledge management, and behavioral studies related to the use of IT.
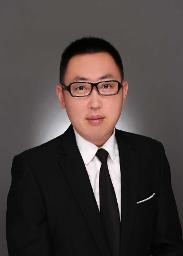
Assoc. Prof. Yunbo Rao
University of Electronic Science and Technology of China, China
Yunbo Rao
received his
B.S. degree and
M.E. degree from
Sichuan Normal
University and
University of
Electronic
Science and
Technology of
China in 2003
and 2006,
respectively,
and Ph.D. degree
from University
of Electronic
Science and
Technology of
China, Chengdu
in 2012, both in
School of
Computer Science
and Engineering
(SCSE). He has
been as a
visiting scholar
of Electrical
Engineering of
the University
of Washington
from Oct 2009 to
Oct 2011,
Seattle, USA. He
has published
over 110
refereed
articles and 10
books. He served
as Chair of the
International
Conference on
Pattern
Recognition and
Artificial
Intelligence
(PRAI 2022). His
areas of
interests
include video
enhancement,
computer vision,
three-dimensional
reconstruction,
virtual reality,
augmented
reality, and
crowd animation.
He also worked
as research
interns at
Neusoft
Inc.during
2004-2008.
Since May.2012,
he joined School
of Information
and Software
Engineering,
University of
Electronic
Science and
Technology of
China.
Currently, he is
an associate
professor at
University of
Electronic
Science and
Technology of
China(UESTC). He
is a supervisor
of Ph.D student
in Dec.2017.